Augmented Collaboration. Emerging Intellectual Synergy
A new look at the idea of the Semantic Web after a quarter century.
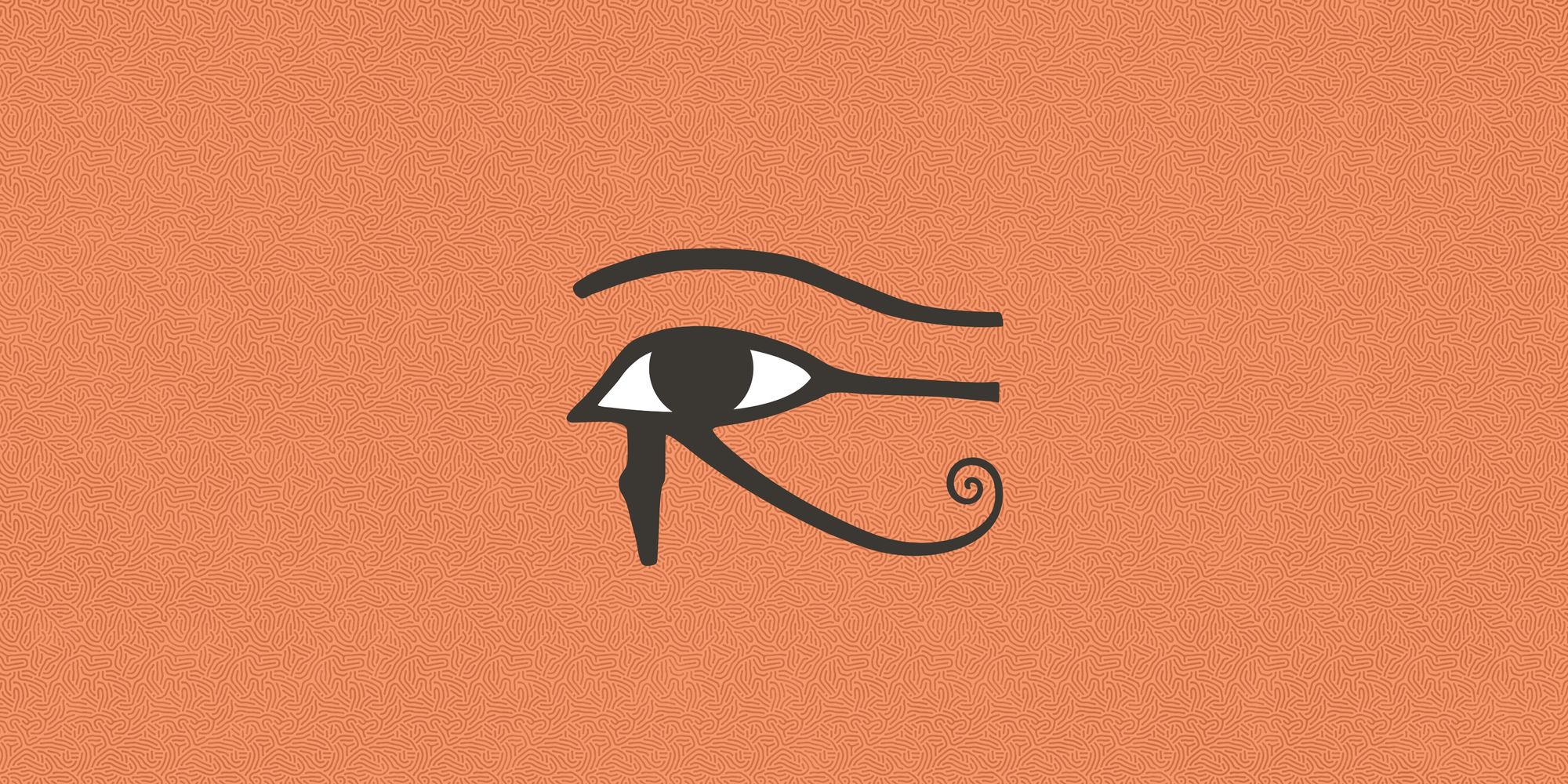
In 2001, Tim Berners-Lee, the creator of the World Wide Web, and James Hendler and Ora Lassila introduced the concept of the Semantic Web in a Scientific American article. The authors described the Semantic Web as an extension of the existing Internet in which each piece of information is accompanied by metadata describing its meaning and relationship to other pieces of information. Such an extension would allow machines to index individual words and fully use the context and meaning of information, opening the way to creating intelligent services that provide instant answers to complex search queries.
Although the idea of the Semantic Web has been applied in various technical fields, the concept did not get the development it deserved. The key problem was the labor-intensive nature of creating and maintaining the metadata layer. In addition, the benefits of the Semantic Web were not as clear, as the value of the Web was determined by both the volume and quality of information. Because the Semantic Web was considered a redundant concept, web data never took an interpretable form - the web today still only serves as an intermediary for information dissemination but in no way an explicit repository of linked data. Instead of a distributed graph, information on the Web is stored as documents and files, each with its structure.
As a result, humanity postponed the idea of a large-scale Semantic Web as a global graph and a means for the evolution of human knowledge until better times. The essential data retrieval mechanism was soon reduced to indexing texts and providing search engines with access to ranked list items rather than providing information. At the same time, analyzing web data involves additional operations such as crawling and parsing, which always work with only some current data cast. The novelty of web technologies, their accessibility and visibility for human interaction with each other eclipsed for a couple of decades the different uses of the Internet - algorithmic processing of up-to-date information not only about the final nodes-objects, but also about the links between these objects, and thus about chains of objects.
After a couple of decades of development of machine learning technologies, it has become possible to solve the same problems indirectly by recognizing relationships from the source text. Large language models allow interaction with data not only at the stage of search engine ranking but also at the stage of data interpretation. In some ways, this solution represents an implementation of Semantic Web tasks, but without the Semantic Web itself - in a less transparent and less robust version, in which resource-intensive tasks are moved from stored markup to the less complete but more abstract markup of language models. In particular, it can be seen that the goals of creating the Semantic Web correlate with the tasks that are being accomplished today with large language models:
- Machine-readable data. Make information on the Internet understandable to humans and machines, allowing computers to automatically process and algorithmically interpret the "meaning" of data.
- Data Integration. Ensure integration of heterogeneous data from different sources, creating a single network of interconnected information.
- Enhanced Search. Provide precise and context-sensitive information search beyond keyword searches.
- Process Automation. Allow software agents to perform tasks automatically using semantic information.
- Creation of a global knowledge base. Formation of a distributed but coherent knowledge system available for use by both humans and machines.
- Logical Inference Support. Enable automatic logical inference based on semantically labeled data.
- Metadata standardization. Develop and implement standards for describing data semantics that allow different systems to "understand" each other.
- Increased interoperability. Improve interoperability between different systems and services on the Internet through a shared understanding of data semantics.
- Personalizing the user experience. Provide more personalized and context-aware services based on a better understanding user needs.
- Support for multilingualism. Facilitate access to information regardless of the language in which it is presented, thanks to semantic links between concepts.
LLM solves one of the key challenges of human-machine collaborative interaction - unrestricted extraction and interpretation of data and the relationships between them, which are available for algorithmic processing. In this publication, we will analyze how the availability of such agents can affect the evolution of collaborative systems and environments, where AI assistants with extensive access to the world's knowledge and digital tools become full-fledged participants in collaborative processes.
AI as a lever for a new approach to collaboration
Business leaders are also recognizing the potential of AI to transform workflows. According to the MIT Sloan Management Review To Be a Responsible AI Leader, Focus on Being Responsible study, 84% of executives believe AI enables their organizations to gain or strengthen competitive advantage. AI is a powerful lever that significantly amplifies specialists' strengths and intuition when solving problems in highly uncertain environments. It is essential to realize that AI does not replace but complements human intelligence. In the AI era, "soft skills" such as creativity, emotional intelligence, and critical thinking are of particular value.
Expanding the cognitive horizon. AI agents in highly collaborative environments do not just complement human intelligence but fundamentally expand the cognitive horizon. For example, the ability to visualize and manipulate multidimensional data opens new horizons for understanding complex systems in science, economics, and sociology. This cognitive expansion increases our ability to solve complex problems and transforms our thinking process, allowing us to formulate questions and hypotheses that were previously beyond our imagination.
Overcoming cognitive distortions. AI systems also serve as a "mirror" for our thought processes, helping us overcome cognitive distortions and identify hidden biases and logical errors. The result is a more objective and rational approach to problem-solving. This process can lead to a profound transformation of individual and collective thinking, creating the basis for more effective and equitable decision-making.
Dynamic epistemology. AI systems can continuously update and re-evaluate the knowledge base on which decisions are based, leading to a more flexible and adaptive approach to understanding the world. This can revolutionize our approach to education and research, where knowledge will not be viewed as a fixed set of facts but as a dynamic, constantly evolving system.
Metacognitive Enhancement. Collaborative participants are empowered to better understand their thought processes and problem-solving strategies. This enhances self-learning and self-development and opens the door to a new level of self-awareness and reflection. We can learn how to solve problems and how to understand how we solve them.
New decision-making models. AI agents can facilitate the development of new decision-making models that combine human intuition, machine analytic abilities, and the harmonious wisdom of the group. As a Harvard Business Review study shows, teams composed of humans and AI outperform both purely human teams and AI-only systems in solving complex problems. This indicates that the future lies in collaborative systems, where human expertise and intuition are harmoniously combined with the analytical capabilities of AI.
Temporal extension of collaboration. AI agents can help overcome the temporal limitations of collaboration by enabling asynchronous collaboration between participants not only from different time zones but even eras, integrating historical data and future projections into the current problem-solving context.
This temporal extension is closely related to the concept of Futures Studies, an interdisciplinary field that explores possible and desirable futures. Within Augmented Collaboration, AI systems can significantly enhance teams' capabilities by analyzing multiple scenarios, integrating heterogeneous data, and identifying weak signals of emerging trends. This approach not only allows teams to work more effectively with the past and present but also to proactively shape the desired future by considering a wide range of possible scenarios and their implications.
Transforming the creative process. AI can significantly transform the very nature of the creative process in collaborative environments. This includes:
- Idea generation: AI suggests unexpected combinations and associations, stimulating participants' creative thinking.
- Iterative design: AI generates multiple solution options, allowing teams to iterate and experiment faster.
- Interdisciplinary Synthesis: AI helps bring together ideas from different fields, fostering innovative breakthroughs at the intersection of disciplines.
- Personalizing the creative process: AI adapts to each participant's style, enhancing their unique creative abilities.
- New level collective creativity: AI acts as an additional "participant" in the creative process, creating a new dynamic in group creativity.
The Power of Diversity: specialization in the age of AI
Several successful web projects involve creating platforms where users with different expertise meet, such as Reddit and Quora, or specialized platforms: Stack Overflow for programmers of all levels, ResearchGate for researchers, Behance for presenting and searching creative works, and ProductHunt for launching new technological products.
In the era of artificial intelligence, the diversity of expertise of collaborative project participants is of particular value. A high level of specialization and deep intuition in a specific field are key success factors. AI acts as a bridge, allowing us to effectively combine the highly specialized knowledge of different experts and provide access to the global knowledge base of humanity.
This approach makes it possible to form new interdisciplinary teams, where it is enough to involve one highly qualified specialist from each required field. AI tools facilitate interaction between experts, overcome language and terminological barriers, and help to quickly find and apply relevant knowledge and experience from other projects and studies.
Support for this concept can be found in several studies and case studies:
- A Science of science study found that interdisciplinary research groups are 17% more likely to publish papers with high citation rates than groups working within a single discipline.
- McKinsey's study The state of AI in 2023: Generative AI's breakout year, found that companies actively using AI to support cross-disciplinary collaboration are 26% more likely to report significant revenue growth compared to companies that do not take this approach.
Key Features of Augmented Collaboration
Unlimited access to interpreted knowledge. As this article was written, OpenAI announced SearchGPT, a new approach to information retrieval that harnesses the power of AI models. Unlike traditional search engines, SearchGPT aims to provide direct answers to questions, supports natural dialog with clarifying questions, and promises up-to-date information with precise citations to sources. The system is capable of delivering visual results, including images and videos. This approach has the potential to significantly change the way users interact with information on the Internet, making search more efficient and personalized.
Interoperability. In a broad sense, interoperability means the ability of different systems, platforms, and tools to interact with each other, exchange data, and use this information without special user effort. In the context of Augmented Collaboration, interoperability is critical, as it combines the capabilities of human intelligence, artificial intelligence, various digital tools, and cyber hubs into a single ecosystem.
Real-time analytics and risk management. ML systems such as Forecast.app analyze project progress data and predict potential problems, greatly enhancing risk management.
Adaptability. Augmented Collaboration tools adapt to each employee's work style, optimizing task scheduling and time management. For example, Microsoft's Cortana and Google's Assistant for Workspace integrate adaptive features into enterprise ecosystems, offering personalized time and task management recommendations.
Completeness of documentation. AI assistants significantly improve documenting meetings, projects, and workflows. For example, Otter.ai offers an AI-powered meeting assistant that creates detailed summaries and action plans. The Fireflies.ai app transcribes meetings and analyzes their content, highlighting key points, actions, and decisions. Notion AI integrates the power of large language models directly into the document creation process, helping users structure information and generate content.
Creative synergy. AI actively participates in the creative process, stimulating innovation and accelerating idea generation. OpenAI's Dall-E 2 platform allows designers and artists to quickly visualize ideas by generating images from textual descriptions. Autodesk's Dreamcatcher uses generative design, allowing engineers to explore thousands of design options based on specified parameters. Adobe Sensei integrates into the company's various products, helping designers automate routine tasks and experiment with new creative approaches.
Introducing AI and new collaborative technologies is leading to significant changes in the social structure of organizations and society. We see a trend towards flattening organizational hierarchies and emerging new AI and data processing roles. The nature of leadership is also changing: leaders must more often act as integrators, bringing together people and AI systems.
Conclusion
The evolution from early experiments with hypertext to the modern era of AI-augmented collaboration reflects humanity's continuous quest for more efficient forms of cooperation. AI, in this sense, does not replace human intelligence but is a cognitive enhancer. ML agents represent a nexus that effectively combines highly specialized knowledge of different experts and access to humanity's global interpretive knowledge base and digital tools. In doing so, the evolution of digital quasi-living systems is accelerated by getting into reality.
Implementing Augmented Collaboration presents several challenges, including ethical issues and the need to develop new skills and adapt organizational structures. Successfully overcoming these challenges will require technological innovation and rethinking how we work, learn, and collaborate. Deloitte's State of Generative AI in the Enterprise (2024) study shows that companies face scaling, trust, and talent management challenges as they move to real-world applications of generative AI. In addition, despite the rapid evolution of AI, some skills remain uniquely human.
A World Economic Forum Future Jobs (pdf) study highlights the importance of soft skills in the age of automation, including creativity, emotional intelligence, critical thinking, adaptability, and ethical judgment. Institutions and companies are beginning to adapt to these demands. For example, MIT has launched Task Force on the Work of the Future, an initiative to study the impact of technological change on the workforce and develop adaptation strategies.
Ultimately, Augmented Collaboration represents a new set of tools and a fundamental shift in how knowledge is created, shared, and applied. This approach can address global challenges, accelerate scientific progress, and create a more inclusive and efficient work environment.